Deep learning segmentation
Object detection using pre-trained algorithms via ONNX models. The object detection algorithms supported can be found under the parameter model type.
See ONNX image segmentation for more information.
TIP
In the analyze tree add the descriptor Segmentation label and select a text file including the names of objects in the ONNX file.
Parameters
Model type
The .onnx model type. Available options are:
Faster R-CNN
YOLO v4
YOLO v5
Onnx file
Select the pre-trained ONNX file for the selected model type.
Source
On which image the ONNX segmentation should be applied. The pseudo-rgb image or a painted prediction image.
Confidence
The confidence level required by the model for an object to be categorized.
Normalize the pixel values
Only applicable to Faster-RCNN
If Normalize and center is used the values will be scaled to 0-1 before the is subtracted and the
used in the division. See below.
No normalization
|
Normalize and center
|
Image dimension order
Only applicable to Faster-RCNN
In which order the input dimensions are:
Width / Height
Height / Width
Output layer to use
Only applicable to YOLOv5
Which type of output layer to use:
Sigmoid layer
Detection layer
Min area
The minimum number of pixels for an object to be included.
Max area
The maximum number of pixels for an object to be included.
If 0 no maximum area is defined.
Object filter
Use an expression to further exclude unwanted objects based on shape.
Standard arithmetic (+
,-
,/
,*
…) and comparison operators (=
,>
,<
…) as well as some mathematical expressions (…) and constant values (
) can be used in expressions.
Properties that can be used for the Expression:
Area
Length
Width
Circumference
Regularity
Roundness
Angle
D1
D2
X
Y
MaxBorderDistance
BoundingBoxArea
For details on each available property see: Object properties Details
Shrink
Takes away x numbers of pixels at the borders of the objects included in images.
Separate
Normal
Can have both separated and combined objects.
Separate adjacent objects
All objects are defined separately.
Merge all objects into one
All objects are defined as one.
Merge all objects per row
All objects per row segmentation are defined as one.
Merge all objects per column
All objects per column segmentation are defined as one.
Max objects
Max number of objects in image, takes the first objects sorted by confidence.
Inverse
✅ Includes the opposite of the sample specified in the Deep learning image model.
⬜ Includes the sample specified from the Deep learning image model.
Link
Only visible when applicable
Link output objects from two or more segmentations to top segmentation. Descriptors can then be added to the common object output and will be calculated for objects from all segmentations.
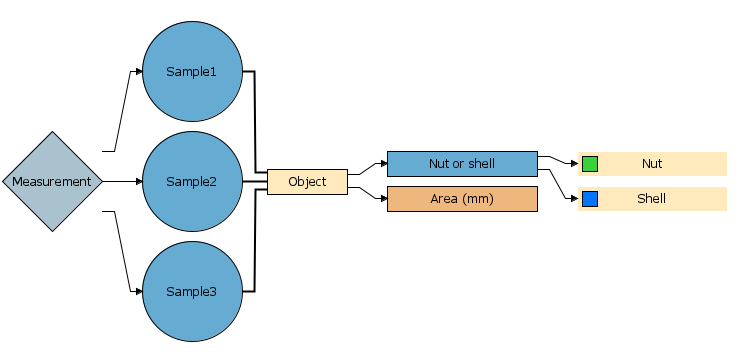
Descriptors after object will be calculated for all three segmentations (Sample1, Sample2 and Sample3)
The segmentations must be at same level to be available for linking.